Can automotive cybersecurity gain inspiration from other fields?
Terence Broderick
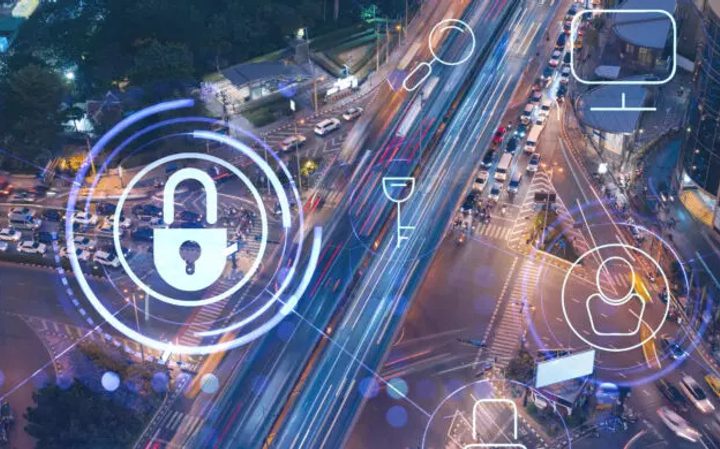
It has been well established that automotive cybersecurity has its
own challenges which pushes it way beyond standard IT security.
The reason for this is the large network of sensors which enable an autonomous vehicle to determine its surroundings and which also, naturally, provides a large number of access points for falsified data representing an attack on the vehicle’s network of sensors.
However, the push towards the proliferation of fully autonomous vehicles goes on and there is a need to look at ensuring these vehicles are secure. One of the biggest challenges is that vehicles need to be secure against unknown types of attack, which places a greater emphasis on a more holistic approach to cybersecurity as the structure of an attack will not always be evident.
Making autonomous vehicles secure with the help of IP
Adversarial machine learning is considered to be a route towards enabling autonomous vehicles to be secure as it enables the development of a holistic, data-driven approach. This field develops algorithms, techniques and methods which protect the machine learning models which drive autonomous vehicles from malicious manipulation and exploitation.
In more technical terms, adversarial machine learning trains models to be able to differentiate between real data, which is being received through a sensor network, and fake data which may be generated by a malicious third party. This means that a model can be trained to respond to sensor measurements which are outside of expected or statistically likely ranges, i.e. those which are indicative of an attack on the model and on the hosting vehicle.
This is a developing field but it does seem worth considering whether innovators in this field could seek inspiration from other fields, such as telecommunications.
Problems with seeking inspiration from other industries
One of the key problems in telecommunications is estimating the likelihood that data is being received in error. In binary terms, that is estimating whether a zero is being received as a one and vice versa. This problem was studied in the specific context of optical communications by Marcuse1 and, as part of the study, the problem was modelled by looking at what are known in statistical inference circles as Type 1 and Type 2 errors. In short, these error types refer to the probability of rejecting a hypothesis which should be accepted. In specific telecommunications terms, this could be estimating a bit transmitted as a 1 as being a 0 (i.e. rejecting the correct hypothesis that the bit is a 1) or estimating a bit transmitted as a 0 as being a 1 (i.e. rejecting the correct hypothesis that the bit is a 0).
Determining whether received data is fake or not is more complicated in that it is not possible to classify the data in terms of zeros and ones but similar logic can be applied when using adversarial machine learning to determine between fake and real data as we can model the fake data as a specific hypothesis and potentially train the model to determine when fake data is being accepted as real data.
Looking outside of a technical field into other technical fields for direction can be indicative of inventive steps in an invention and this will help the argument for patent protection. However, there may also be pitfalls in using the work of others when innovating.
If you are innovating in automotive cybersecurity by applying the data analytics techniques which have worked in other fields then please get in touch as it may be that you have a patentable invention.
Please contact Terence Broderick if you would like to discuss this further.
1 D. Marcuse “Derivation of analytical expressions for the bit-error probability in lightwave systems with optical amplifiers” Journal of Lightwave Technology Vol. 8, Issue 12