‘Conscious’ AI — how close are we to replicating the human brain?
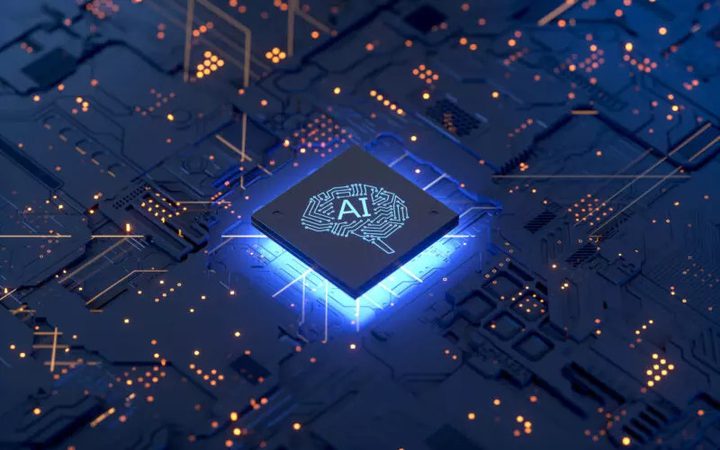
From The Matrix to Avatar, humans have long dreamt of the possibilities unlocked by replicating and interfacing with our brains.
Now, with the likes of ChatGPT taking the world by storm, many are wondering just how far AI technology can go. Could we soon be able to recreate the human brain, or even outperform its ability to understand, learn and create?
What is the brain — and why is it special?
The human brain is a finely tuned clump of biological material. It has adapted over millions of years to parse precisely the type of sensory information deemed to be selective for survival in whichever environment we (and our evolutionary ancestors) found ourselves during this period of adaptation.
We know that sensory information came in the form of at least five main input methods: visual and auditory cues within a rather narrow band of the electromagnetic and auditory spectra (visible light, audible frequency range), along with tastes, smells and touch (of the range and sensitivity determined by evolutionary circumstance to be most important for day-to-day survival).
The capabilities of the brain have extended beyond this largely mechanical input-output relationship between the body and the surrounding environment. We link inputs to understanding and learning, and furthermore to generating thoughts, emotions, opinions and perspectives, as well as generating and developing concepts in a creative manner. To this day, such achievements by the human brain can only be described nebulously, using vague ideas like ‘consciousness’ and ‘self-awareness’.
Why replicate the human brain?
The potential benefits of replicating the human brain’s capabilities range from the relatively academic and philosophical (such as uploading human consciousness) to the more pragmatic and commercial (such as computing extremely difficult tasks efficiently).
This inevitably hints at a desire to not only mimic human capabilities, but to outperform them. Such benefits are limited only by the imagination (whether real or artificial) and speculating on the effects and consequences of such revelations is beyond the scope of this article. But what about the current status of efforts in the field?
The complexities and nuances behind the meaning of ‘consciousness’ notwithstanding, it’s often overlooked that for industry, attempts at mimicking the pure sensory input-output capabilities of the brain represent a solved, or at least solvable, problem — particularly in the case of visual and auditory (and to some extent, touch) input. Artificial systems have long been able to far surpass the sensory capabilities of the human eye and ear, vastly extending detection distance and spectral sensitivity in modern-day instruments. Together with recent advances in artificial olfactory sensors (electronic noses) and gustatory sensors (electronic tongues), it’s reasonable to assume that human sensory systems have been (or shortly will be) adequately replicated or surpassed.
However, anything beyond merely detecting this raw sensory input towards understanding and learning based on what is seen or heard (at the level performed by the human brain) requires an attempt at approximating the brain’s learning processes.
The brain-power problem
These learning processes are highly efficient, with the human brain using only around 10 to 20W of power (yes — less than an average lightbulb), compared with modern (classical) supercomputers, which actively require tens of megawatts and occupy entire buildings.
The difference in efficiency can largely be explained by the hardware constraints of classical computers compared with the biological make-up of the brain. Studies estimate that the brain has between 100 and 200 billion neurons, with each of these having around a thousand or more connections to other neurons, providing over a trillion connections on average for a human brain. These connections allow neurons to combine their efforts in the creation and storage of memories for the purpose of ‘learning’. Replicating such learning in a classical computer therefore presents a significant hardware challenge.
Efforts to mimic how such memory formation takes place are long established in the form of neural networks. Neural networks provide a software solution to the process of learning, but often continue to run across hardware limitations when attempting to approximate brain-level functionality.
It’s becoming clear that the problem of replicating the human brain represents a mix of difficult hardware problems and difficult software problems.
The state of innovation
From a software perspective, the brain effectively functions in a manner approximating a type of neural network known as a recurrent neural network (RNN). However, on current-generation computational hardware, RNNs run into many hurdles with scalability, particularly in specific types of learning tasks such as natural-language processing (NLP).
This has led to efforts to tailor neural network approaches to specific types of task to obtain a greater level of performance. More recent focuses on NLP-related tasks use a type of neural network usage called a ‘transformer’ (such as the well-known Generative Pre-trained Transformer 3 — commonly known as GPT-3 — from Open AI).
Other task-specific types of neural network exist to aid in approaching brain-level learning functionality. For example, machine vision-related tasks are typically performed using convolutional neural networks (CNNs) which typically involve traversal of various filters or ‘kernels’ across a still image, pixel-by-pixel, to perform tasks such as to determine (or rather estimate) the content of the image.
For such software efforts to scale in performance using modern hardware constraints, more powerful hardware is often required, which inevitably results in increasing the number of transistors on a computer chip (thus increasing power usage).
By both adapting task-specific software approaches and increasing hardware capability to scale such approaches, these solutions move further and further away from the known functioning of how the brain operates.
Attempts at more closely approximating the structure of the brain through software look to mimic the complex connectivity structure of the neurons within the human brain or the ‘connectome’, including the production of ‘neuromorphic’ neural networks.
From a hardware perspective, the brain makes use of electrochemical exchange at synaptic interfaces in order to transfer information. Approaches to adapt hardware to more closely mimic synaptic interactions include ‘neuronal chips’, which make use of physical, rather than virtual (software-implemented) neurons similar to the biological after-hyperpolarization (AHP) neurons involved in memory formation. These neuronal chips aim to provide more efficient algorithmic processing than what’s possible on standard computing hardware — specifically for memory access and development. Other recent techniques look to directly connect lab-grown brain tissue (specifically, stem-cell derived ‘brain organoids’) with 3D microelectrode arrays in an attempt to create a living tissue/computer hybrid system termed ‘organoid intelligence’ for achieving a similar data processing efficiency to that achieved by the human brain [Smirnova et al 2023, Frontiers in Science].
These issues facing both the hardware and software solutions highlight the importance of combined hardware and software co-design methodologies in achieving the goal of mimicking brain functionality.
Patent protection for AI innovations
Innovation continues to move at pace in this area, as we can see from the number of patent application filings, which have shown exponential growth over the last decade. It’s clear that the primary focus of efforts to date have been software related and due to the limitations of current computer capabilities, more effort may be required in the hardware area.
From a patentability perspective, the inclusion of hardware solutions — whether alone or as a complement to software solutions — can aid patentability in jurisdictions such as the UK and Europe, where an emphasis is placed on hardware contributions or effects having real-world technical effects beyond the running of an algorithm per se. Therefore, while a mix of hardware and software solutions is most likely to be required in mimicking brain functionality with AI, this mix also benefits from the improved likelihood of patentability in many such jurisdictions around the world.
How close are we to replicating the human brain?
With almost 150,000 AI-related patent applications filed worldwide in 2021 [Center for Security and Emerging Technology via AI Index Report, 2022], the work is well underway.
By more closely approximating the human brain by adapting current hardware and software approaches, we may come to better understand the concept of ‘consciousness’ — which may in-turn reveal previously unforeseen advancements in AI and ways in which it could be integrated into our everyday lives.
There are, of course, those who remain sceptical as to the feasibility of the effort to precisely mimic human brain functionality in general. Opinions along these lines include that computers are inherently incapable of performing tasks that the human brain performs on a daily basis, such as ad-hoc category construction tasks. Others say that the brain acts merely as part of a more complex whole, and that a brain without a body simply lacks this essential context for developing and maintaining a sense of ‘consciousness’.
Whatever your perspective, if you’re working at the cutting edge of AI technology, get in touchto arrange a free initial chat about your IP with our specialist AI team.